Introduction
Metabolic syndrome is an escalating global health issue, largely driven by rising obesity rates and sedentary lifestyles.1 The primary characteristics of MetS encompass several medical conditions, including glucose intolerance, hypertriglyceridemia, abdominal obesity, low HDL levels and hypertension.2 This syndrome represents a collective variables that significantly elevate the likelihood of evolving T2DM and cardiovascular diseases.3
Adipose tissue significantly contributes to the initiation and advancement of metabolic disorders by synthesizing numerous adipocytokines. Among these, hyperleptinemia is particularly plays significant involvement in the onset of diabetes, hypertension, coronary heart disease and stroke. 4, 5 Circulating leptin, an adipokine primarily released by white adipocytes, serves as a multifaceted molecule involved in various physiological processes. These include appetite suppression, glucose utilization, increased energy expenditure, regulation of adipogenesis, and the progression toward insulin resistance.6
Emerging research is shedding light on various theoretical mechanisms that contribute pivotal roles in patients with Metabolic Syndrome. For instance, some researchers have demonstrated a significant association between body adiposity and leptin levels, indicating a correlation with obesity and contributing to insulin resistance, thereby increasing the risk of developing type 2 diabetes (T2DM). Moreover, hyperleptinemia has been identified as an autonomous risk factor for the onset of heart diseases and MetS.7, 8 Notably, research has revealed that women with Cardiac Syndrome X exhibit higher circulating leptin levels compared to healthy controls, even after controlling for body mass index.9 Furthermore, studies have observed reduced circulating adiponectin levels but elevated leptin levels, alongside an increased leptin/adiponectin ratio in CSX subjects compared to controls. In obese individuals, increased circulating leptin levels often correlate with greater adipose tissue accumulation. Hyperleptinemia is speculated to contribute significantly to insulin resistance.10 Moreover, females have higher leptin levels compared to males when these levels are adjusted for body fat percentage.11, 12
The hypotheses collectively suggest that circulating leptin plays a significant role in Metabolic Syndrome. A meta-analysis was performed to quantify the strength of the relationship between circulating leptin levels and MetS in adults. Furthermore,meta-regression analysis was performed to investigate other influencing factors in MetS. This research could help in early identification and intervention to reduce the incidence of MetS-related diseases.
Materials and Methods
Search methodology
A systematic medical literature search was conducted using MeSH (Medical Subject Headings) terms such as 'Serum Leptin', 'Leptin', 'Metabolic Syndrome', and 'Metabolic Disease', with the assistance of trained librarians. The search encompassed articles published prior to June 2023 and spanned electronic repositories (PubMed, Scopus, Web of Science, Google scholar, Cochrane Library and Embase), employing pre-defined search strategies. Titles and summaries of the relevant studies were meticulously reviewed followed by a thorough evaluation of full texts of related studies to autonomously identify further eligible studies.
Prerequisites for study
Prospective studies fulfilling the following inclusion criteria were considered for inclusion in this study.
Full research articles focusing on Leptin levels in Metabolic syndrome.
Studies including human participants aged between >18 years and <75 years.
Studies that provide leptin central tendency and dispersion for cases and controls both.
Conversely, studies not adhering to above mentioned criteria were excluded:
Reviews or case reports.
Animal studies.
Gray literature (unpublished literature).
Studies combined with other diseases.
Two studies were ruled out due to inadequate reporting of data for control (healthy) and MetS groups.
Data extraction
Data from selected study were collected employing a uniform data extraction template, focusing on approach and dependent variables as follows:
(a) author’s name and publication year; (b) type of biological samples (c) country of study; (d) number of participants; (e) study parameters; (6) laboratory parameters; (7) anthropometric parameters;(8) assay methods; and (9) mean or median concentration of leptin for control and patient groups.To organize extracted data Excel 2021 and software EpiData 3.0 was used.
Statistical analysis
In these meta-analyses, 14 selected studies were considered depending on sample size, study method, and year of publication shown in Figure 1. The analysis of statistical data was performed using the software MedCalc® version 22.00. The study compared the mean leptin levels and standard deviations between cases and controls. The proportional weight of each study was assigned according to its sample size. An independent sample t-test was applied to each study, and a 95% CI was drawn. The combined estimates of mean and standard deviation for cases and controls were calculated using a weighted average method, where weights were based on the sample size of each study. The standardized mean difference (SMD) and their respective 95% confidence intervals were also computed for each study. A combined estimation for all 14 studies was performed.
Table 1
The table is prepared with mean and standard deviation (SD) of variables contributing factors forMetS from selected studies.
Study |
Material |
Country |
No. of Samples |
BMI |
Mean Age |
FPG |
SBP |
DBP |
HDL |
TG |
WC |
Methods |
Goguet-Rubio et al., 2017 13 |
Serum |
US |
16 |
48.5 ±3.73 |
38.5 ±2.2 |
111.6 ±7.2 |
136.3 ±4.9 |
85.75 ±1.5 |
43.4 ±1.8 |
141.86 ±9.4 |
NR |
ELISA |
Surpriya et al., 2018 14 |
Serum |
China |
19 |
NR |
65 ± 11 |
122.4 ± 23.4 |
159 ± 17.2 |
83.9 ± 12.5 |
38.6 ± 7.7 |
221.4 ± 40.0 |
92.6 ± 10.2 |
ELISA |
Gasier et al.,2016 15 |
Serum |
US |
33 |
30 ± 3 |
22 ±39 |
89 ±8 |
133 ± 12 |
82 ± 8 |
44 ± 9 |
160 ± 120 |
95.9 ±13.2 |
ELISA |
Anna Szczepańska-Szerej et al.,2017 16 |
Serum |
Poland |
59 |
27.5 ±5.3 |
67.8 ±10.2 |
125.1 ±34.8 |
NR |
NR |
47.2 ±16.2 |
154.6 ±67.3 |
NR |
ELISA |
Yun-Jung Bae et al., 201317 |
Serum |
Korea |
198 |
28.0 ± 3.4 |
46.5 ± 11.5 |
108.5 ± 12.5 |
135.5 ± 15.7 |
78.7 ±13.3 |
42.6 ± 12.1 |
212.2 ± 114 |
95.8 ± 7.6 |
ELISA |
Tseng PW et al., 2017 18 |
Serum |
Taiwan |
45 |
27.61 ±2.85 |
67 ±4 |
138.0 ± 13 |
151.5 ±19.7 |
85.0 ±10.7 |
44.0 ±10.9 |
141.7 ±60.40 |
93.53 ±6.60 |
ELISA |
Chia-Chu Liu et al., 2017 19 |
Serum |
Taiwan |
234 |
27.6 ± 9.2 |
56.9 ± 6.0 |
109.8 ± 25.5 |
131.2 ± 12.1 |
82.6 ± 8.6 |
42.2 ± 8.5 |
170.9 ± 86.4 |
90.6 ± 7.0 |
ELISA |
Somaye Yosaee et al., 2017 20 |
Plasma |
Iran |
51 |
31.4 ± 3.7 |
37.4 ± 6.5 |
116.2 ± 37.3 |
121.2 ± 11.7 |
80.8 ± 9.1 |
50.3 ± 8.3 |
266 ± 203 |
105 ± 8 |
ELISA |
Li Hsuan Wang et al.., 2017 21 |
Serum |
Taiwan |
30 |
27.02 ±3.60 |
72.97 ±4.78 |
122.5 ± 58 |
134.7 ±21.9 |
71.63±9.50 |
45.07±9.55 |
157.30±61.52 |
98.1 ±9.98 |
ELISA |
Olesia M. Bochar et al. ,2021 22 |
Serum |
Ukraine |
90 |
32.24 ±0.52 |
57.49±1.07 |
NR |
168.2 ±2.28 |
107.2 ±0.66 |
44.08 ± 1.93 |
188.6 ± 11.5 |
95.87 ±1.33 |
ELISA |
Arthur SC et al., 2017 23 |
Plasma |
Brazil |
157 |
30.9 ± 1.3 |
63 ± 2.6 |
107.4 ± 6.6 |
142 ± 3.0 |
82 ± 1.1 |
43 ± 2.2 |
142.4 ± 19.2 |
100 ± 13 |
ELISA |
Abhishek G et al. ,2010 12 |
Serum |
India |
186 |
27.22 ± 5.07 |
28.32 ± 5.94 |
101.1 ± 17.3 |
132.4 ± 11.4 |
88.46 ± 7.8 |
41.98 ± 4.66 |
142.6 ± 36.0 |
86.35 ± 14.0 |
ELISA |
Vinod W et al. ,2016 24 |
Serum |
India |
63 |
27.22 ± 4.16 |
49.7 ±5.32 |
106.6 ±32.4 |
129.2 ±5.19 |
84.11±5.14 |
39.78±7.22 |
151.56±56.23 |
NR |
ELISA |
KW Liang et al.,2015 25 |
Serum |
Tiawan |
15 |
28.57 ± 1.72 |
53.8 ± 4.31 |
111.4 ± 6.9 |
NR |
NR |
41.31 ± 2.30 |
155.3 ± 21.8 |
NR |
ELISA |
Figure 2
Forest Plot illustrating Leptin Levels between MetS Patients and Controls. Each datum represents a selected study, with the marker size corresponding to the count of participants in a particular study. The diamond indicates the overall effect size ofleptin levels from the selected studies.
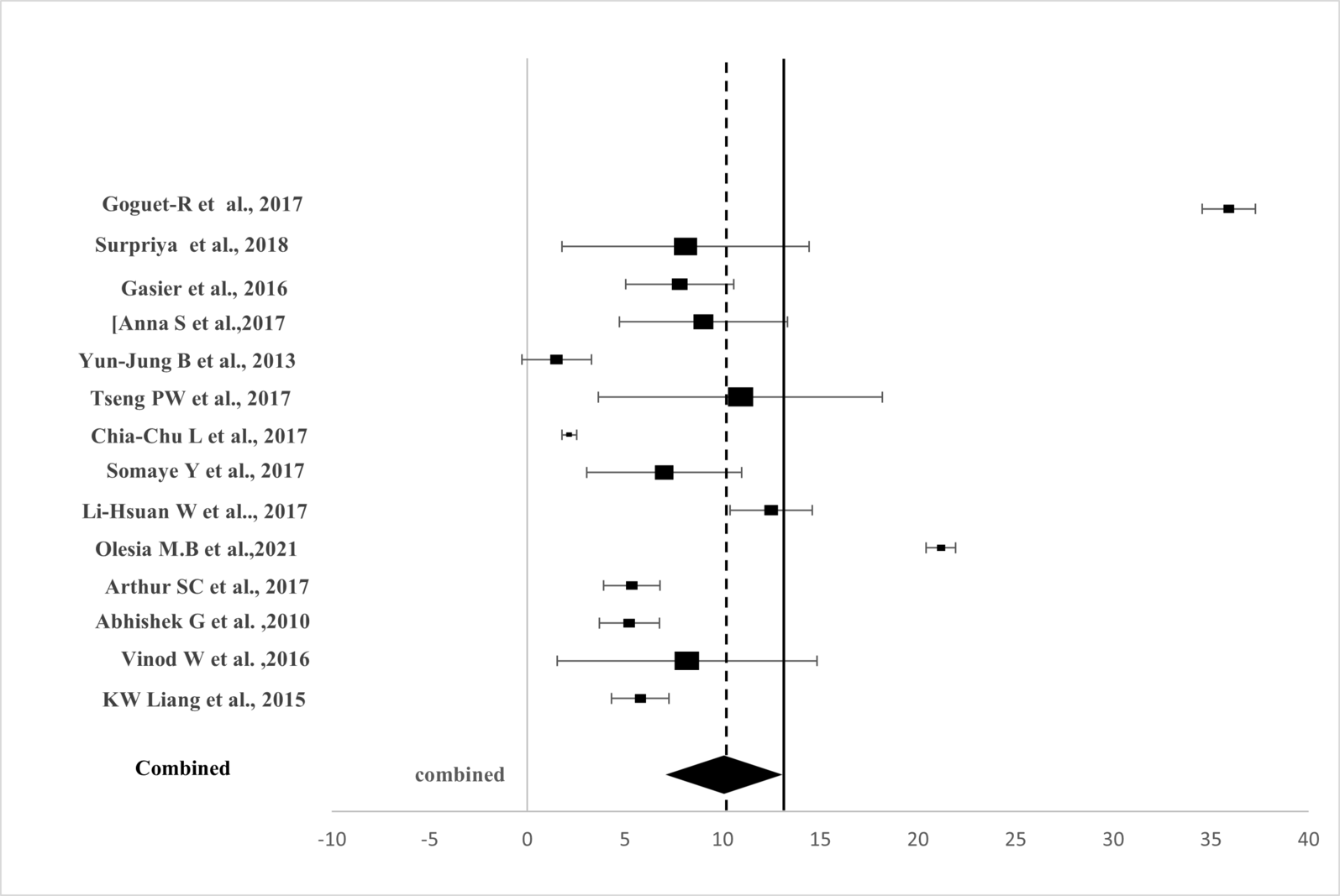
Table 2
Assessment of Heterogeneity. The figure illustrates the leptin concentrations between Metabolic Syndrome (MetS) as N2 and controls as N1, combining studies to produce an estimate with a significant p-value less than 0.001, 95% CI for each.
Study |
N1 |
N2 |
Total |
SMD |
SE |
95% CI |
T |
p |
Weight (%) |
|
Fixed |
Random |
|||||||||
Goguet-Rubio et al., 2017 13 |
16 |
24 |
40 |
16.754 |
1.900 |
12.908 to 20.599 |
|
|
0.084 |
2.27 |
Surpriya et al., 2018 14 |
19 |
20 |
39 |
0.814 |
0.327 |
0.151 to 1.476 |
|
|
2.83 |
8.01 |
Gasier et al.,2016 15 |
33 |
20 |
53 |
1.856 |
0.319 |
0.946 to 2.226 |
|
|
2.98 |
8.04 |
Anna Szczepańska-Szerej et al.,2017 16 |
59 |
99 |
158 |
0.678 |
0.168 |
0.346 to 1.010 |
|
|
10.74 |
8.50 |
Yun-Jung Bae et al., 201317 |
108 |
91 |
199 |
0.236 |
0.142 |
-0.0443 to 0.517 |
|
|
14.99 |
8.55 |
Tseng PW et al., 2017 18 |
45 |
19 |
64 |
0.811 |
0.280 |
0.252 to 1.370 |
|
|
3.88 |
8.18 |
Somaye Yosaee et al., 2017 20 |
51 |
51 |
102 |
0.688 |
0.202 |
0.286 to 1.089 |
|
|
7.41 |
8.41 |
Li Hsuan Wang et al.., 2017 21 |
30 |
32 |
62 |
2.965 |
0.366 |
2.233 to 3.697 |
|
|
2.27 |
7.86 |
Olesia M. Bochar et al. ,2021 22 |
90 |
50 |
140 |
9.721 |
0.607 |
8.521 to 10.921 |
|
|
0.82 |
6.74 |
Arthur SC et al., 2017 23 |
157 |
79 |
236 |
1.011 |
0.145 |
0.725 to 1.297 |
|
|
14.39 |
8.54 |
Abhishek G et al. ,2010 12 |
186 |
204 |
390 |
0.676 |
0.104 |
0.471 to 0.880 |
|
|
28.02 |
8.61 |
Vinod W et al. ,2016 24 |
63 |
63 |
126 |
0.431 |
0.179 |
0.0759 to 0.785 |
|
|
9.45 |
8.47 |
KW Liang et al.,2015 25 |
15 |
39 |
54 |
2.363 |
0.376 |
1.608 to 3.117 |
|
|
2.15 |
7.82 |
Total (Fixed effects) |
872 |
791 |
1663 |
0.848 |
0.0551 |
0.740 to 0.956 |
15.407 |
<0.001 |
100.00 |
100.00 |
Total (Random effects) |
872 |
791 |
1663 |
2.028 |
0.333 |
1.376 to 2.681 |
6.096 |
<0.001 |
100.00 |
100.00 |
Because of differences in populations and outcome criteria, a random-effects model (REM) was employed to detect combined estimates from selected studies.13 Figure 2 and Table 2 show SMDs and 95% CIs for individual studies and combined estimates. It pooled 95% CIs and ORs to assess internal exposure impact on outcomes. SMDs and their 95% CIs were combined using Cohen’s d to evaluate MetS and leptin concentration relationships.
Heterogeneity across meta-analyses was estimated by employing both the Cochrane Q test and the I² statistic. High heterogeneity was defined as I² greater than 75%, moderate as 25% to 75%, and low as less than 25%. 14 A sensitivity analysis was performed to evaluate the impact of excluding specific studies on total outcomes. Additionally, publication bias was assessed using the Egger funnel plot and linear regression test for studies with ten or more reporting meta-analysis results. 15 All statistical analyses were two-tailed, and significance was evaluated at a p-value threshold of less than 0.05
Observation and Results
Literature review
Figure 1 illustrates the article selection process for this meta-analysis. Initially, 1,492 articles were selected from major database searching tools like pubmed, web of science, cochrane library and scopus. Ultimately, 14 articles published within the last decade met the inclusion criteria and were selected for meta-analysis.
Study features
Metabolic syndrome was characterized depending on the NCEP-ATP-III criteria, which requires meeting any 3 out of 5 conditions: (1) Waist circumference: ≥102 cm for males or ≥88 cm for females, (2) Triglycerides: ≥150 mg/dL or taking cholesterol-lowering drugs, (3)Fasting plasma glucose ≥100 mg/dL or using glucose-lowering agents (4) Systolic blood pressure (SBP): ≥130 mm Hg or diastolic blood pressure (DBP): ≥85 mm Hg, or both or using medications for hypertension and (5) HDL-Chol: <40 mg/dL in males or <50 mg/dL in females. 14
rovides a comprehensive overview of the 14 eligible studies, detailing subject demographics such as material used, geographic region, participant numbers, BMI, and mean age; laboratory parameters including fasting plasma glucose (FPG), SBP, DBP, HDL, triglycerides, and waist circumference; and details regarding circulating leptin samples, including assay methods. In total, these studies compared circulating leptin levels across 1,171 controls and 1,106 MetS patients.
Comparative analysis
Figure 2 presents the forest plot depicting SMDs derived from the meta-analysis using a random-effects model. Table 2 illustrates significantly elevated circulating leptin levels in cases compared to controls with SMD = 0.84, 95% CI: 0.74 to 0.96, Z = 3.10, p < 0.001. Significant heterogeneity was observed among the studies (I² = 96.74%).
Table 3
Meta-regression analysis of studies on circulating leptin and MetS parameters
Table 3 summarizes the findings of the meta-regression analysis. No significant differences were observed in BMI, SBP, DBP, HDL, and triglyceride levels. However, inclusion of Age and Fasting Plasma Glucose (FPG) in the meta-regression model revealed that FPG significantly contributed to the sources of heterogeneity (95% CI: 0.91 to 1.8, p = 0.009), along with Age (95% CI: 0.98 to 1.19, p = 0.047).
Figure 3
Non-linear regression analysis illustrates the relationship between FPG values and SMD results derived from data in each study.
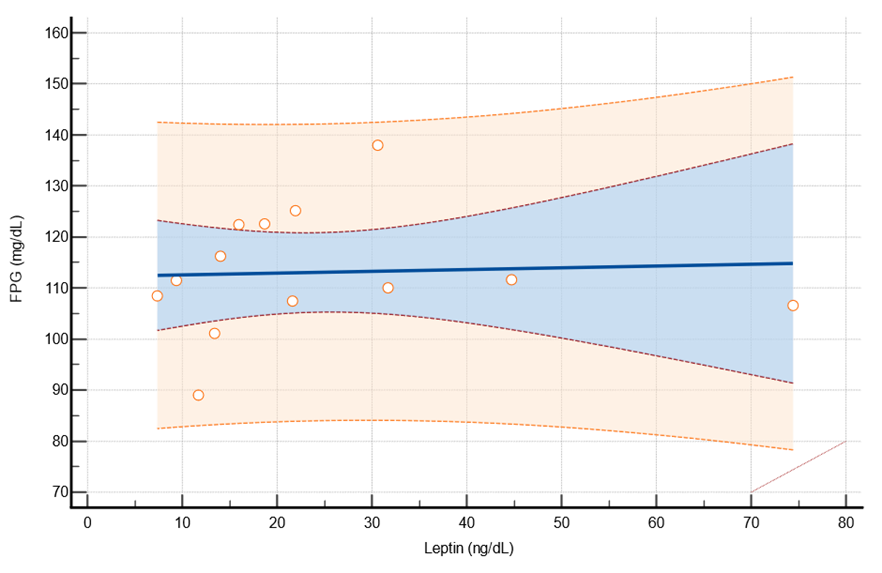
Figure 3 displays the findings from non-linear regression analysis between Fasting Plasma Glucose (FPG) and Leptin Standard Mean Difference (SMD). A positive relationship was observed between FPG and Leptin, with a significance level of p-value less than 0.001.
Figure 4
The logistic regression analysis predicts the likelihood of Metabolic Syndrome (MetS) using rising FPG levels from the studies as independent variables.
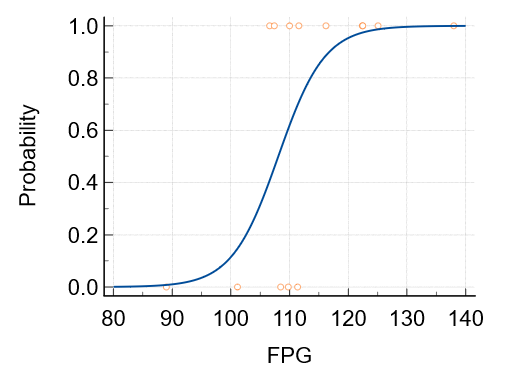
The sensitivity analysis demonstrated that the diagnostic ability of leptin in MetS was excellent (AUC = 1.000, p < 0.001), and for FPG it was also high (AUC = 0.852, p = 0.004). The Egger funnel plot for circulating leptin levels appeared symmetrical, while Egger's test did not indicate publication bias (p value = 0.0019, 95% CI: 3.92 to 13.17).(Figure 4)
Discussion
Metabolic Syndrome (MetS) is a multifactorial disease with complex pathogenic mechanisms, but central obesity and insulin resistance (IR) play crucial roles in its development. 1 This meta-analysis shows significantly higher circulating leptin levels than control with positive correlation, suggesting that elevated leptin levels may increase the risk of developing MetS. Furthermore, the study observed a slight trend towards a higher risk of MetS with increasing leptin levels. However, the meta-regression showed significant heterogeneity, largely explained by fasting plasma glucose (FPG). A positive linear relationship was observed amongst SMD of leptin and FPG, indicating that FPG significantly influences the regulation between circulating leptin and MetS. The study also examined data from different age groups to assess whether age variations impact circulating leptin levels.
Numerous studies have indicated that circulating leptin could potentially impact the risk of diabetes and cardiovascular disease. The link between T2DM and circulating leptin reveals significantly higher levels in T2DM individuals compared to controls. This study proposes that elevated circulating leptin levels may contribute to disruptions in the metabolism of glucose and lipids in individuals diagnosed with MetS. 11 Although it shares similarities with T2DM and CVDs, the circulating leptin in MetS is plays more complicated role and requires further investigation. Moreover, among women with MetS, serum leptin levels exhibited a significant positive correlation with HOMA-IR (p Value < 0.001) along with metabolic risk factors, alongside negative correlations with FBS, TG, and HDL-c. In adult women from North India, circulating leptin showed significant associations with insulin resistance, hyperlipidemia and alteration of metabolic risk factors. 11
Studies by Supriya et al.14 suggest that individuals with central obesity may experience more severe inflammatory conditions in Metabolic Syndrome (MetS), while research by Goguet-Rubio et al. 13 indicates that adipokines can act as biomarkers for detecting pathophysiological changes in the body, facilitating early diagnosis. Gasier et al.'s study on obese volunteers found that 30% of those meeting MetS criteria face a 3 to 7 times greater risk of developing diabetes and heart diseases. 15 Additionally, leptin levels were notably associated (p value < 0.001) with ischemic stroke, suggesting its as a potential biomarker for assessing the risk of cardioembolic stroke in patients with Metabolic Syndrome. 16
The results indicate that circulating leptin levels were elevated in Metabolic Syndrome (MetS) patients compared to controls across all age brackets.26 However, meta-analysis in adults showed significantly higher heterogeneity, suggesting that circulating leptin activity or bioconversion mechanisms could be compromised in adult patients diagnosed with Metabolic Syndrome (MetS). Additional research is required to elucidate the molecular mechanisms that underlie these variations in leptin levels among the patients of different age with Metabolic Syndrome (MetS).
Multiple studies have demonstrated a positive relationship between MetS scores or the presence of MetS and leptin levels (p < 0.05).17, 18, 19, 21, 20 Leptin levels are markedly elevated in overweight children compared to controls, indicating potential impairment in metabolic processes.22 The leptin/adiponectin ratio (LAR) examined by Catharina et al. suggests it may act as a dependable biomarker for hypertensive individuals at risk of developing MetS, with an odds ratio of 4.13 (p = 0.01).27 The circulating levels of the adipokine leptin also demonstrate significant associations with insulin resistance indices in individuals, regardless of whether they have cardiac or metabolic syndrome X. 25
Leptin abnormalities, such as hyperleptinemia or leptin resistance, are pivotal in Metabolic Syndrome (MetS). Diagnosis depends on glucose and lipids levels, blood pressure, BMI and waist measurements, indicating pathological conditions. Early MetS detection is critical due to its association with heightened disability and mortality risks.13 Asians face increased T2DM and obesity-related disease risks at lower BMIs than Europeans,5 likely linked to elevated blood glucose levels seen in MetS, contributing to heightened circulating leptin. Biomarker use in early MetS diagnosis can aid disease management and prevent irreversible complications.
Limitations
Limitations of our meta-analysis and meta-regression study include several factors. Firstly, while a regression relationship was found between circulating leptin and Metabolic Syndrome (MetS), all analyzed studies were case-control, preventing causal inference. Future research should incorporate prospective cohort studies to better explore this link. Secondly, Several eligible studies failed to adjust for potential variable confounders. Thirdly, there is considerable variability in how circulating leptin levels relate to MetS across studies, indicating methodological or population differences. Future investigations should aim to study this association in more homogeneous populations using consistent methodologies. Lastly, language bias is restricted to the English language. These limitations highlight the importance of interpreting our findings with caution.
Conclusions
The concentration of circulating leptin was notably elevated in Metabolic Syndrome patients compared to controls, and there was a suggestive trend indicating a potential increase in MetS risk with higher leptin levels. Furthermore, fasting plasma glucose (FPG) appears to exert a regulatory influence regarding the association between circulating leptin and MetS. These findings underscore the importance of assessing circulating leptin levels to understand their role in the pathophysiology of MetS. The findings from this meta-analysis may facilitate early identification, the exploration of new biomarkers, and the uncovering of potential therapeutic targets for addressing Metabolic Syndrome (MetS).